Chapter 1 Theoretical Foundations
One of the defining human characteristics is the ability to use language in its lexical and combinatorial richness. The study of language acquisition has been a traditional locus of our search to understand the nature of this ability. What allows human children to acquire a language has been the subject of one of the historical “great debates,” in which highly diverse proposals about the architecture of the human mind and the nature of human uniqueness have been formulated. Does language arise from domain-specific adaptations for syntactic structure (Chomsky 1981, 2014)? Or does it arise from a combination of environmental input and sophisticated, general-purpose learning mechanisms (Elman et al. 1996)? Two poles have traditionally emerged in this discussion: from domain-general empiricist proposals to domain-specific nativist proposals, as illustrated in Figure 1.1.
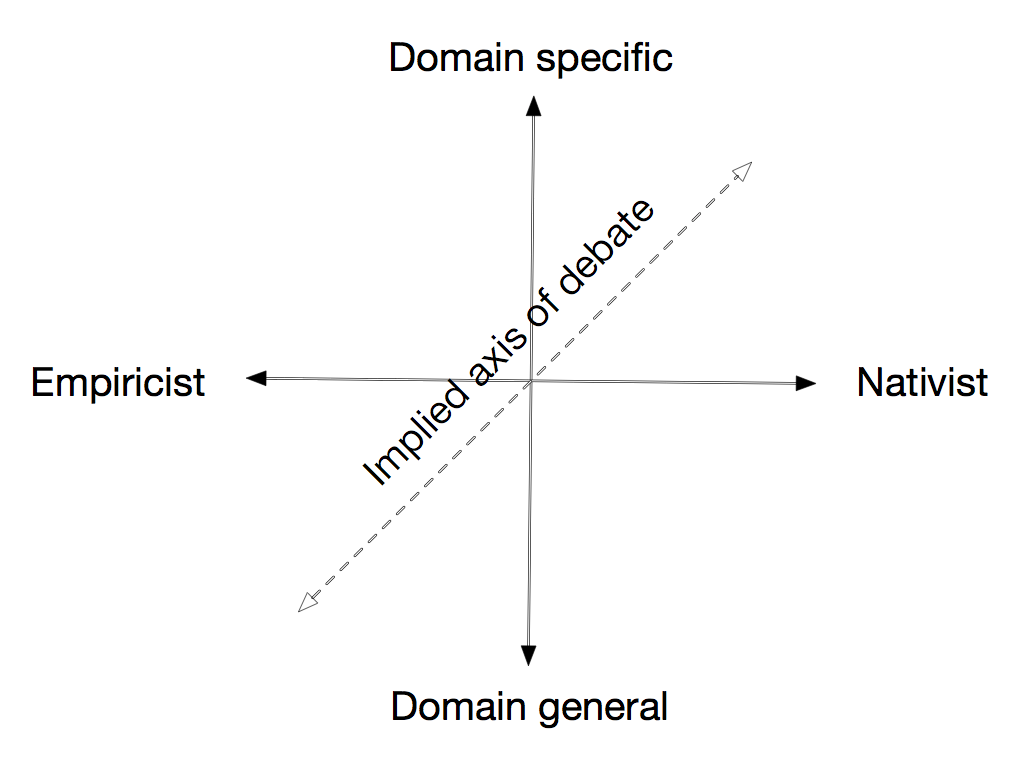
Figure 1.1: Schematic of the space of theoretical debates around language acquisition.
In this chapter, we begin by presenting the perspective from these poles but contend that they are rarely helpful in the practice of understanding the scope and course of early language learning. Instead, we argue for the development of theories that describe the scope and course of language learning as a whole, as well as its quantitative variation across children, languages, and cultures. These concerns lead us to frame our own study in terms of a set of distinct theoretical issues: capturing consistency and variability; drawing connections across timescales of learning; and the notion of process, rather than content, universals. We end by discussing the relation of our theoretical stance to others and the role of replication and larger datasets in building quantitative theories.
1.1 The picture to date
Nativist proposals regarding language acquisition have typically been tightly focused on syntactic development, assuming that children’ phonology, morphology, and lexicon are learned from their input. Within the domain of syntax, the emphasis is on the complexity of the grammatical structures that young children appear to show mastery of (e.g., Crain and Thornton 2000). To the extent that these are rare in children’s environment, this rarity could suggest an innate foundation for children’s knowledge (e.g., Legate and Yang 2002). The type of proposals that are on offer within this space often include “principles and parameters”-type theories, in which languages share a set of syntactic principles that govern syntactic combination but vary on a relatively small set of parameters that control how these structures vary (Baker 2005; Yang 2002).
These proposals are challenged by cross-linguistic variation in syntactic abstractions (Evans and Levinson 2009), by the character and scope of children’s syntactic generalizations (which are often tied to specific lexical structures; Tomasello 2000), and by evidence of early input-sensitive learning and generalization both within specific domains (e.g., Meylan et al. 2017) and in artificial language tasks (Gómez and Gerken 1999). Such proposals also tend to downplay the inherent variability that characterizes language learning across individuals (Bates, Bretherton, and Snyder 1988; Bates et al. 1994), focusing instead on the universals or commonalities that exist across children. While nativist proposals acknowledge that individual variation exists, variation is not typically used as a lever into the process of acquisition, existing in a “theoretical vacuum” (Bloom 2002, 52). Far greater emphasis in this tradition is placed on how language acquisition works in the general case.
In contrast, empiricist proposals for language learning emphasize the ability of children to learn structure across domains, the richness of the distributional input that children are exposed to, and their ability to create appropriate abstractions from structured input. One important component of these proposals is children’s general statistical learning abilities (Saffran, Aslin, and Newport 1996; Fiser and Aslin 2002). Work in this tradition demontrates that even quite general statistical tools can recover some aspects of linguistic structure across a variety of domains when they are applied to sufficient data (Redington, Crater, and Finch 1998; Frank, Bod, and Christiansen 2012; Elman et al. 1996). Further, general statistical learning abilities are likely extremely useful for learning sound structures (Feldman, Griffiths, and Morgan 2009) and vocabulary (Smith and Yu 2008). Thus, one strength of more empiricist proposals is their ability to deal with a broader set of acquisition phenomena than syntax alone.
Empiricist proposals regarding linguistic structure are sometimes referred to as “emergentist” or “constructivist” accounts. Such accunts have as a primary challenge that, even at an early age and with limited input, children sometimes show evidence of abstractions that encode key aspects of linguistic competence. For example, young children show some systematicity in the word order of their productions even in the absence of structured input (Goldin-Meadow and Mylander 1983; cf. Bowerman 1973). Further, at least by some time in their third year, typically developing children appear interpret the arguments of novel verbs (Gertner, Fisher, and Eisengart 2006) and show evidence of category structure for syntactic categories such as determiners (Yang 2013) and structures such as the dative (Conwell and Demuth 2007). These results are sometimes intepreted as suggesting that while general learning mechanisms are necessary for language acquisition, they are not sufficient – especially in the domain of syntactic structure.
The “great debate” between these viewpoints is philosophically appealing, but has also led to a polarization of the field of language acquisition. Typically researchers work in their own siloed traditions (empiricist or nativist), and focus on individual phenomena that do not make contact with one another – a classic version of Kuhn (1970)’s “paradigms.” Research in the nativist tradition often focuses on particular syntactic phenomena that are largely neglected in the empiricist tradition (e.g., the “optional infinitive,” Wexler 1998; but cf. Freudenthal, Pine, and Gobet 2010). In contrast, research in the empiricist tradition has often used artificial language learning tasks that are argued not to reflect the underlying structural properties that are claimed to be innate (e.g., Lany and Saffran 2010; cf. Yang 2004). Empiricist theories are also more likely to recognize the causes and consequences of individual variation in rates of learning, including potential sources of that variation that arise from variation in the circumstances in which children learn and the opportunities they have for engaging with language in a meaningful way (e.g., Huttenlocher et al. 1991; Hoff 2006; Weisleder and Fernald 2013). By focusing on different paradigms and phenomena and theorizing using distinct vocabularies, these traditions make limited contact with one another. One prominent language development conference has traditionally featured two distinct plenary talks, one from each of these two different traditions – a clear sign of polarization.
To be tested, proposals must make specific predictions. But these theories are frameworks, and few proposals within these frameworks can be said to generate testable and clearly competing predictions, even within a specific langugae and linguistic domain. Any individual observation typically cannot be said to be inconsistent with any but the absolute strongest nativist or empiricist position. Computational models have been an important method for allowing proposals to be instantiated to the degree that they can make testable predictions. In practice, however models typically end up less differentiated than framework rhetoric suggests. In order to get off the ground in performing a particular empirical task, theories must often help themselves to generous amounts of both innate structure – in the form of structured inputs from social, cognitive, or perceptual domains – and statistical learning abilities (Roy and Pentland 2002; Alishahi and Stevenson 2008; Frank, Goodman, and Tenenbaum 2009; Frank et al. 2010; Yang 2004).2
Further, when nativist and empiricist viewpoints do make differing predictions, they are often in phenomena that – from a bird’s eye, or even a parents’ eye, view – are relatively trivial in the general course of language development. This observation is especially true in the domain of syntax. Abstraction debates have played out in the acquisition of the definite determiner “the” (Valian 1986; Pine and Lieven 1997; Yang 2013; Meylan et al. 2017), auxiliary inversion (Pullum and Scholz 2002; Legate and Yang 2002), and the use of anaphoric “one” (Akhtar et al. 2004; Regier and Gahl 2004; Lidz, Waxman, and Freedman 2003), for example. These highly-specific and complex phenomena are occasionally observable in the children of linguistically-trained parents, but even the closest observer would be forgiven for being more compelled by watching the increasingly creative and complex ways that children interpret, use, and play with language, rather than the occasional syntactic slip. Further, all of these phenomena reflect a broader historical argument that syntactic structure is the heart of the uniquely human language faculty (Chomsky 1957), and that other aspects of language tend to be shared with other species (Hauser, Chomsky, and Fitch 2002) and are therefore somehow less interesting and less critical as objects of study.
From an evolutionary perspective, however, syntax is far from the only unique or notable feature of human communication (Pinker and Jackendoff 2005; Tomasello 2010). The nature and range of children’s communicative gestures, the variety of sounds used in human languages, and the diversity of lexical items available to speakers all are relatively unprecedented, especially within the primate lineage. And these observable aspects of language – as well as the emergence of syntactic structure more broadly – are some of what makes the broad course of language acquisition striking from the perspective of a researcher, clinician, or a parent. As observers, we notice the first communicative signals, the emergence and rapid growth of vocabulary, the beginning of the productive combination of words, increases in the length and complexity of utterances, and the patterns of error and overgeneralization that remain in early childhood. Moreover, there is considerable evidence for continuity across these domains (e.g., Bornstein and Putnick 2012; Tsao, Liu, and Kuhl 2004; Bates, Bretherton, and Snyder 1988; Cristia et al. 2014). Children’s earliest gestures and sounds relate to their oral language comprehension and production. And, these in turn relate to later skill in using language as a tool for learning, through both auditory (or visual, in the case of sign language) and written modalities.
These broader patterns of language learning are the natural focus of investigations like ours that use parent report to learn about children’s language. Parents are attentive and accurate observers of communicative gesture, vocabulary, and word combination. But without linguistic training they may not even notice subtleties like non-productive determiner use, auxiliary inversion, or anaphoric “one.” Further, investigations of the broader dynamics of acquisition using parent report can, in many cases, make productive contact with the rich literatures on early communication, speech perception (e.g., Kuhl 2004), word learning (e.g., Bloom 2002; Snedeker 2009), and grammatical productivity through verb structure (Fisher et al. 2010). While debates over the nature of syntactic knowledge and abstraction have raged, thee subfields of language acquisition that focus on these more directly observable phenomena have prospered. Research in these subfields makes at most limited contact with broad questions of nativism and empiricism, in part because they deal with phenomena that are language-specific – sounds, lexical items, grammatical constructions – and hence that children must learn from their input. The question is then about the mechanisms and constraints that guide this process of learning, rather than about any posited universal or innate content (even at the level of abstractions).
1.2 Making progress
A unifying framework is necessary in order to move beyond great debates. What should a unifying theoretical framework for language learning look like? In spite of the critiques above, we still believe in the importance of the search for core, universal aspects of language learning that elucidate the process by which children acquire this uniquely human ability. Yet we also believe that the sort of theory that describes such universals will likely look radically different from its historical antecedents. Below and in the remainder of this chapter, we sketch some aspects of what such a theory might look like and how this vision connects to our present investigation.
We start with the observation that any universal of language acquisition is likely to be a statistical or quantitative universal – we refer to these as “consistencies.” The variation across the world’s languages is such that very few properties will be truly invariant (Evans and Levinson 2009). Further, we are unlikely to be able to access the kinds of samples that would allow us to make claims of universality (one estimate suggests that strong statistical support for claims of universality require evidence from more than 100 languages from diverse families; Piantadosi and Gibson 2014). Thus, we will talk about the relative consistency and variability of particular phenomena rather than any sorts of absolutes, and we expect any empirically-grounded theory will do so as well.
In addition, language learning takes place at the timescale of years. A parent’s responses on a CDI form provide a global snapshot of a child’s language at a particular point in time, rather than demonstrating the operation of a mechanism or principle. Substantial reconstruction is necessary to understand how processes operating over seconds – for example, online statistical learning (Aslin and Newport 2012) or pragmatic inference (Bohn and Frank 2020) – would result in particular structures accreting over time in the vocabulary. Thus, consistencies we observe are at best the basis for abductive inferences about underlying mechanisms.
These consistencies are consistencies in the learning of vocabulary and constructions, rather than syntactic rules. Words and constructions must be learned from data. Thus any putative universals identified in our investigation must not be “content universals” that specific particular grammatical rules or linkages. They must be “process universals” in the sense that they specify mechanisms or processes that unfold over time and operate over children’s interactional input in ways that produce the observed consistencies.
Before we begin spelling out these ideas in more depth, we note that there are several important precedents for this line of argument. First, harkening back to Bates, Bretherton, and Snyder (1988) and Elman et al. (1996), our proposal is a shift from a focus on consistencies in representational content to a focus on consistent learning mechanisms. Second, Clark (1977) distinguish between “process” and “product.” In the language of this distinction, our work in this book aims at uncovering processes that lead to the products that parents observe and report on the CDI. Finally, these “process universals” are likely to be similar to the “operating principles” discussed by Slobin (1973, 1985), although Slobin’s initial idea was that these would be elements of the Chomskian Language Acquisition Device, and hence likely language-specific. In contrast, as we will see below and throughout our narrative, in our analysis many apparent process universals seem to be driven by non-linguistic factors like children’s general statistical learning abilities as well as the ways they allocate their attention to the world around them.
1.3 Variability and consistency
An starting point for our analysis is the observation by Bates, Bretherton, and Snyder (1988) that what “hangs together” in development can provide clues about the architecture of the underlying language learning mechanisms. We think of these correlations – the statistical instantiation of “hanging togeether” – as providing targets for theorists. A successful theory can gain support by providing an account for these correlational observations as its explananda. Crudely put, if a theory posits that some aspect of language acquisition is universal, it should be relatively more consistent in our data.
What are the units over which we should compute variability and consistency? We refer to these as “signatures” – loosely, measurements that can vary across populations. In practice, a signature can be the output of any analysis, with the simplest being vocabulary size or variability itself (as in Chapter 5). Signatures are linked to particular theoretical goals by arguments about the validity of an analysis – for example, the argument of Bates et al. (1994) that the over-representation of nouns in early vocabulary is a meaningful dimension of variation between individuals. A signature for our purposes is thus an analysis that yields a set of numbers. In nearly every chapter of the book, we define one or several signatures whose variability we can measure.
Different sources of variability provide different sorts of evidence. One sense of the notion of “universal” that dates to early generative syntax is the notion of typological invariance – invariance across languages (Greenberg 1963). Following this general idea, in the majority of the book we focus on variability in some signature across languages. The implied inference is that consistency across languages in some signature implies that the signature results from a mechanism (more on inferences about mechanism below) that is independent of the language being learned and the context in which it is learned.
When we assess the variability of some signature across languages, we are also examining variability across datasets; hence, many things vary that are not the target of our inference. Different datasets are constructed by different researchers with different goals. They use different instruments with different items, and different lengths, and different categorical composition. These instruments are administered to different samples, with different sampling strategies. The nature of the administration – the instructions, whether the form is given online or on paper – is sometimes different as well.
Thus, when a particular response appears to be consistent, we can say a fortiori that none of these sources of variation appear to have affected the consistency of the response. (Or at very least that if they have, they have canceled each other out in a highly non-random way). When variability does occur, we cannot make the opposite inference. Thus, variability has many explanations, but consistency tends to point us towards a single inference.
We focus on cross-dataset variability as the primary source of variability in this book. We refer to this variability throughout as cross-linguistic variability, though in fact there are a number of caveats that must be stated. First, many things vary between datasets far beyond language (as noted above). And second, some datasets represent the same language in different dialects (e.g., Australian and British English). Some even reflect the same language and dialect, measured using two different instruments (e.g., the two Beijing Mandarin datasets). In some cases, we can even leverage these parallels to help us rule out alternative explanations. There are three reasons we focus on cross-dataset variability.
First, datasets vary so much that – assuming this variation is somewhat random – claims of consistency are that much stronger when they emerge from this sort of data. Imagine, counterfactually, that all of the instruments we used had exactly the same structure and item set, and all were administered identically. Certainly, this lack of variation would make our life easier in a number of ways when making quantitative comparisons between datasets! But, it also then means that these consistencies would be confounded in our data – particular item sets (plausibly) or administration instructions (somewhat less plausibly), or both, could be the source of an observed consistency in the data. In contrast, while the messiness and inconsistency of the data in the Wordbank dataset make many aspects of our analysis much harder, it actually increases the strength of the inferences we can draw when – despite this – we see some phenomenon emerge with striking consistency.3
Second, the genesis of the investigations documented in this book was in part the observation that several phenomena that we examined were strikingly consistent across languages. For example, the gender effects shown in Chapter 6 were much more consistent than any of us thought (being at the time ignorant about the previous literature in this particular area; Eriksson et al. 2012). Empirically, when we browsed the Wordbank dataset, we found a lot to look at that was both surprising and interpretable when we examined well-known signatures across languages. Thus, our motivation was in part circular: the emergent success of the cross-dataset approach made us curious about what comparisons were possible.
The final reason we consider cross-dataset variability as our primary lever is a negative one. The obvious competitor as a source of variability is variation across individuals. We examine this variability to some extent in Chapters 4 and 5 and dive in even more extensively in Chapter 15. While we document substantial and stable variability across individuals (echoing Bates et al. 1994), this variability empirically proves to be less of a lever into theoretical issues of interest than we would hope. One reason for this is data-related – we have far more cross-sectional than longitudinal data in the Wordbank dataset – and hence, we cannot track stability and change over time as easily or powerfully as we would like (but cf. Chapter 13). Further, we have very few additional measures on most children in the dataset (beyond the broad demographic features, such as maternal education, that we report on in Chapters 6 and 9). In addition, as we show in Chapter 15, though there is some reliable stylistic variation between children, much of the apparent variability in children’s style of language learning can be traced to variation in learning rate. Thus – and in contrast to the exciting emergent conclusions from cross-linguistic variation – for us, individual variation appears to be a less powerful theoretical lever.
Nevertheless, individual variation across individuals does exist and it is robust. Indeed, in Chapter 5, we show remarkable consistency across languages in the extent to which there is variability across individuals. We remain optimistic that continuing to explore the extent and consistency of this variability will to provide a window into the universal processes that guide learning for the mythical “model” child, as well as define the upper- and lower-limits on typical development. Our pessimism about the promise of theorizing around individual variability is one that is limited only to the current dataset. In the longer term we are optimistic about the lessons that can be learned from individual variation in language learning.
In Chapter 16, we bring together estimates of variability of individual signatures from each of the earlier constituent chapters. We combine these into a single, data-driven continuum from consistency to variability, and use this continuum to drive speculations about the sorts of mechanisms that would produce the observed pattern of data. We next consider some theoretical constraints for these speculations.
1.4 Process universals
1.4.1 Preconditions
Imagine we were to uncover an aspect of language development that was completely consistent across languages. (Surprisingly, as we will see in Chapter 16 there are some!). What could we then infer from this observation? Not much, it turns out. The observed regularity could be due to different sources in different datasets or it could be uninteresting from a theoretical perspective.
First, even if the consistency were interesting, any inference from it will always be abductive – an inference backwards from observation to cause. These abductive inferences will always be under-constrained and tentative, thus they will always be at best empirically-grounded speculations that should be brought together with other data to make a test. In some sense, this is the fundamental caveat governing our entire enterprise here. Our research design is correlational and so definitive causal inferences are not possible.
Inferences can go wrong even within this more limited correlational paradigm. For example, we might observe that, across languages, a particular word (or more precisely, the set of word forms corresponding to the same concept) was always produced earliest. It could be the case that the word happened to be learned earliest in some languages because it was short and easy to pronounce, while in other languages, it was learned early due to a high frequency of usage in the input. This example illustrates the difficulties of reverse inference from consistency. Similarly, we could observe that a certain distributional form always described children’s vocabulary estimates, across languages. This regularity could be due to the operation of the central limit theorem rather than any interesting or substantive mechanism that we might be interested in as psychologists.
These problems mean that we need to have two (somewhat informal) conditions on the consistencies that we posit. First, we need to consider the possibility of multiple routes to the same observed consistency. To the extent that observed regularities are specific and surprising, it will be less likely that there are multiple routes across different languages to observing the same thing. Second, for any potential causal story that we posit, we need to be able to posit a plausible or interesting causal story that does not generate the observed regularity. The tightness of this comparison with a counterfactual governs the strength of the inference.
1.4.2 The nature of the processes
Suppose a consistency we identify meets the conditions we describe above: it is sufficiently surprising that we do not see a parsimonious story for how the data for different languages could have been generated by different processes, and there are close counterfactual circumstances in which this consistency would not emerge. Further, in our example, suppose we have a sufficiently large and diverse set of languages and cultures represented in our dataset such that we can justify using the title “universal” rather than the more descriptive and limited term “consistency.” We can then imagine trying to constrain the nature of the sort of universal that could give rise to this type of consistency.
What can we say about such putative universals? First, to the extent that they arise from reports of children’s vocabulary, they cannot be universals of content. Words are learned from a set of specific interactional circumstances in a child’s history (e.g., the trip to the zoo where a giraffe was seen for the first time). Thus, there is no viable sense in which possible universals for learning of this sort could be content universals; the English word “giraffe” is not innately given. For this reason, we describe these putative universals as “process” universals: they relate to the process by which each individual extracts generalizations from their own idiosyncratic experiences.
Further, since these processes are fundamentally learning processes, they operate at the timescale of moment-to-moment interactions (Frank, Goodman, and Tenenbaum 2009; McMurray, Horst, and Samuelson 2012). Using the CDI, we observe the accretion of vocabulary and linguistic competence over the course of millions of these interactions (see e.g., Dupoux 2018 for estimates). This mismatch in timescales makes inferences about the nature of the processes even trickier. We are studying “macro-economic” indicators about the global outcomes of the learning system, hoping to connect these to the micro-economic dynamics of individual “markets” for specific words.
Yet, from the perspective of the language learning literature, there are still obvious candidates for the sort of process universals we are talking about. The general idea of “statistical learning” is one (Saffran, Aslin, and Newport 1996; Aslin and Newport 2012; Saffran and Kirkham 2018). From a very early age, children are sensitive to regularities in their sensory environments and track statistical associations between elements in these environments. Concrete examples of these mechanisms in action include the tracking of syllable-to-syllable conditional probabilities (Saffran, Aslin, and Newport 1996) and the tracking of word-referent correspondences (Smith and Yu 2008), but in principle these mechanisms are likely operating over every level of representation present in early language (Shukla, White, and Aslin 2011; Dupoux 2018).
This viewpoint is quite consistent with the general perspective of “language as skill learning” advocated by Chater and Christiansen (2018). Since all aspects of language learning are subject to the general dynamics of repetition and practice, we should expect to see effects of word frequency pervasively throughout our data – and indeed we do. These effects manifest themselves directly in Chapter 10 as well as perhaps more indirectly in the demographic variation we study in Chapter 6.
Processes of statistical learning operate in a social and pragmatic context (Bohn and Frank 2020). Statistical learning processes operate over social input that includes information from social partners (C. Yu and Ballard 2007). In addition, it is likely that statistical learners take into account the nature of the social context in the inferences that they make (Frank, Goodman, and Tenenbaum 2009; Shafto, Goodman, and Frank 2012; Frank, Tenenbaum, and Fernald 2013). Such social inferences are the focus of much research (including our own). But because they are among the most ephemeral aspects of in-the-moment interactions, they will probably be visible only very indirectly in our data (for example as they are affected by sociodemographic variation, e.g. in Chapter 6).
Processes of generalization are also strong candidates for process universals. Because the CDI does not query the details of lexical meaning, we cannot detect conceptual generalization principles, though these are likely at work (Xu and Tenenbaum 2007), but we can look for some evidence of morphosyntactic generalization mechanisms. The nature of these generalization mesomechanisms is highly controversial (for all the reasons discussed above), but every account of learning requires some type of generalization from specific lexical items to syntactic constructions or morphological rules (Tomasello 2003; Yang 2016). We discuss constraints on these generalization processes in Chapter 13.
In addition, a number of processing factors might lead to processes that are universal. At the same time as children’s language abilities are growing, a variety of core aspects of cognition are undergoing developmental change as well. Children’s general speed of processing is changing (Kail 1991; Frank, Lewis, and MacDonald 2016) – including changes in memory (Ross-sheehy, Oakes, and Luck 2003; Rovee-Collier 1997), attention (Colombo 2001), and executive function (Davidson et al. 2006). Processing factors also influence the speed and efficiency with which children can comprehend words in real time, linking both to developmental change and individual differences that are stable and meaningful and that extend beyond language (Fernald and Marchman 2012; Marchman and Fernald 2008; Marchman and Dale 2017). Although much of the developmental literature on these cognitive constructs focuses either on infancy or the preschool years – because one- and two-year-olds are hard to measure with standard cognitive psychology tasks – the assumption is that these processes are developing continuously throughout the period we focus on here. These developmental changes mean that the processes that we describe are not static but – inasmuch as they draw on these capacities – are themselves a moving target.
Finally, it is important to note that process universals need not be internal to the child.4 Instead, they could be universals of interaction between children and their caregivers. Specific signatures could emerge from these processes in just the same way as they could from processes internal to the learner. To take a concrete example, the timing of turn-taking in conversation is one such proposed interactional universal (Stivers et al. 2009). It is entirely possible that some of the specific consistencies in the content of children’s early vocabulary (Chapter 8) emerge from consistencies in children’s early environments, including universal features of what children and their caregivers talk about and why.
1.4.3 Alternatives
In Chapter 16, we will examine the empirical support for the claim of consistent signatures in language learning across languages. Building on these data, our further claim is that these consistencies are supported by universal processes. It is helpful to consider what the alternative is to this position. Perhaps the most important alternative view is that the process of language acquisition is specific and particular, rather than universal. Two prominent sets of particulars ground this alternative.
The first is the vast semantic and syntactic variation across the world’s languages. For example, as illustrated by Slobin (1996) and others, languages vary dramatically in the ways that they assign semantic content to verbs. If the semantic partition of verbs led to large-scale differences in the timeline or mechanism of acquisition, we might see systematic differences in the predictors of age of acquisition for verbs in these languages, yet we do not. Further, languages are more and less morphologically complex; while the most highly morphologically-complex, polysynthetic languages are not represented in Wordbank, we do have data from relatively less complex (Mandarin) and more complex (Russian) languages. If morphosyntax were relatively more or less important in the acquisition of particular languages, we might expect radical differences in the noun bias, the grammar-lexicon correlation, or the predictors of age of acquisition across languages. Yet, we do not observe these. Of course, there is always room for finer-grained predictions – with more detailed predictive models and better typological coverage, perhaps we will discover such variable morphosyntactic signatures. Our point here is merely that – to the extent that we observe consistencies, neither morphosyntactic nor semantic variability across languages dominates the process of vocabulary acquisition.
The second set of language-specific particulars that might lead to variance across languages is the vast cultural variability across the communities represented in the Wordbank data. Our data contain both “individualist” and “collectivist” cultures (Markus and Kitayama 1991; Nisbett et al. 2001) as well as both “loose fit” and “tight fit” cultures (Gelfand et al. 2011). To the extent that parenting differs across these cultures – and there is good evidence that it does (e.g., Bornstein 2013) – we should see variance in the trajectory of language learning. For example, it would be quite reasonable to predict that the female advantage in vocabulary acquisition might vary as a function of cross-national gender biases (e.g., as measured in Nosek et al. 2009). Yet it is strikingly consistent overall (presaging the conclusions in Chapter 6), again arguing that – at the broadest level, at least – variable cultural factors do not dominate other processes in the acquisition of vocabulary.
1.5 Replication and theory-building: Conclusions
In this chapter, we have sketched a bit of what we see to be the unique theoretical contributions of work with a much larger dataset than is usual in developmental language acquisition research. In a nutshell, doing this work at scale allows for the identification of sources of variability in the “signatures” of language learning. What these signatures are is a matter for further development – each chapter will describe and motivate the particular signatures that it includes. Further, the consistency of these signatures can provide a motivation for positing process universals that underlie the emergence of these signatures (pending the caveats stated above). We return to the general picture of language learning that emerges from our study in Chapter 17.
One final note about the theory that emerges from the work we do here. One set of concepts that is subsumed in our interest in consistency is that theory be supported by observations that are reproducible, replicable, and robust (Munafò et al. 2017). A theory of consistencies is, again, a fortiori all of these. If a particular characteristic can be shown again and again across individuals, samples, and languages it is replicable. Indeed, one view of our enterprise is that its impact is fundamentally in the consolidation of knowledge through unifying – replicating – previous work.
Crudely put, we have compiled all of the CDI data that we could, and all of the CDI analyses, and executed the cross of analyses and datasets. The project of this book is thus in some sense a cross-linguistic replication study. And so, when we state that some phenomenon is consistent across languages, it is by definition replicated – but it is additionally robust to a number of different procedural decisions (such as the design or administration of the CDI form) that end up varying widely in our data.
Finally, this work is also fully computationally reproducible – the analytic conclusions we draw here based on a set of open data and code that can be rerun to create the figures and tables in the manuscript. This characteristic alone does not guarantee their correctness, but at a minimum their provenance is known.
Moving onward from the high-level theoretical framing we have given in this chapter, our next chapter introduces some of the practical foundations of our study, including the reliance on parent report and the nature of the Wordbank project.
The research on the nature of inflectional morphology – the “past tense debate” – is one place where computational models have played a foundational role in instantiating theoretical claims about innateness and representational structure (e.g., Rumelhart and McClelland 1986; Pinker and Prince 1988; Plunkett and Marchman 1993, 1991, 1996; Marcus 1995; Marchman 1997).↩︎
Of course, we also consider the confounds that do remain. In particular, confounding related to the parent report structure of the CDI is a major risk that we discuss at length in Chapter 4 and also in Chapter 17.↩︎
Our proposal diverges here from earlier accounts like Slobin (1973)’s operating principles, which were assumed to be learner-internal.↩︎